Advances in Autoencoders for Healthcare
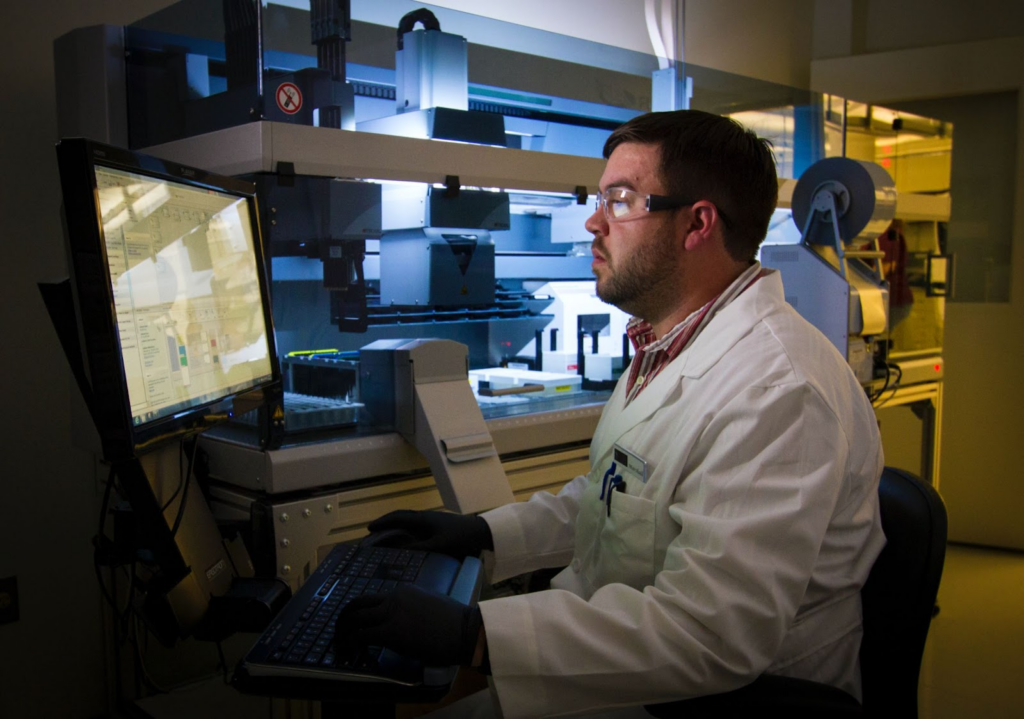
Introduction
Autoencoders, or self-learning codes, help solve problems with data classification and understanding. These codes are used extensively in the computer field, but have recently gained traction in healthcare as well. In this blog article, we will explain what an autoencoder is and how it can be used to solve several different problems in healthcare. AE can effectively identify the operating conditions in which the equipment is being used. The results recorded in the experiments show high accuracy and F1 scores, which makes the technique applicable in real scenarios. AE largely overcomes competing methods in both supervised and unsupervised scenarios. The method is robust to the chosen window size.
Autoencoders in healthcare settings
Autoencoders are an innovative form of artificial intelligence (AI) used in several important fields of research. These include communication, defense, and healthcare. Autoencoders are machine learning algorithms that can effectively interpret and analyze data. The basic idea behind an autoencoder is to map input data into a series of hidden states. The final state of the autoencoder is the output resulting from the mapping process. The process repeats itself until it reaches a desired level of accuracy. This allows for fast and effective data analysis, which is extremely useful in healthcare settings.
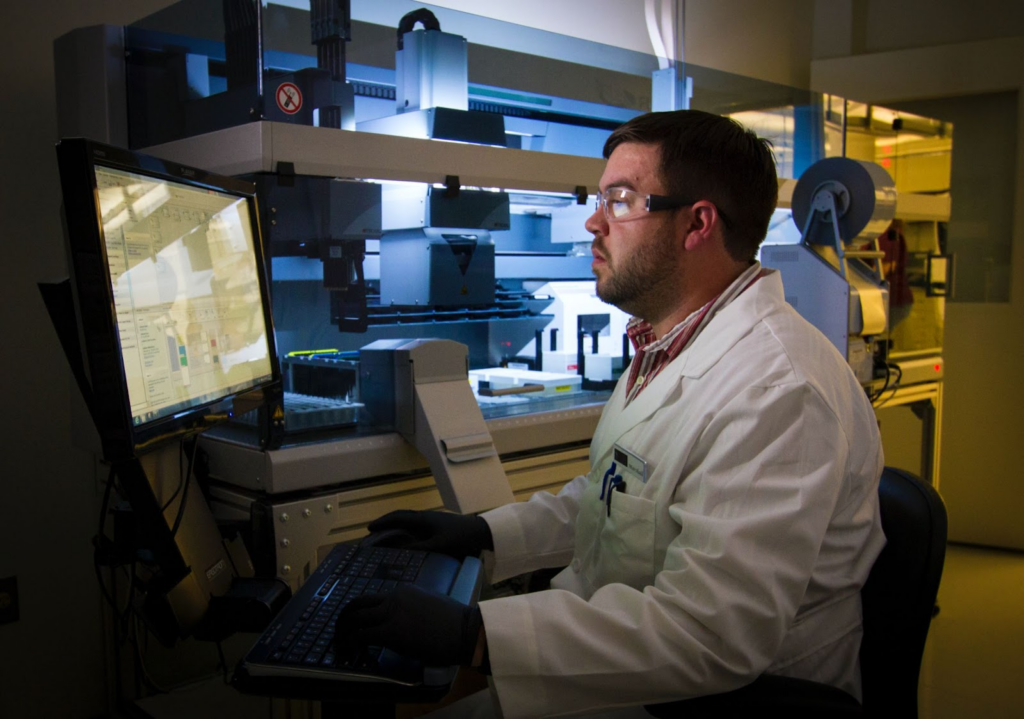
Autoencoders have many applications in these fields because they can effectively train machine learning algorithms to detect heart conditions and Discovering Patient Phenotypes. They can also help healthcare providers identify at-risk patients and target treatment. Healthcare providers are using autoencoders to improve patient care and lower healthcare costs. In addition, autoencoders can be used to encode and decode data with error correction capabilities for secure communication. Oftentimes, medical data is extremely complex and can be extremely difficult to interpret.
An autoencoder can help patients and doctors by converting medical data into human readable text. This makes it possible to quickly find relevant information and understand what the data means. Several researchers used autoencoders to train machine learning algorithms for cardiovascular surveillance in 2017. These included computerized dynamic recccordialte (CODA), heart rate variability analysis and transatlantic voyages in a fugue state for systolic pressure management diagnosis via deep neural network (DANVAS). Researchers used this statistical data to evaluate the health of the cardiovascular system of US army personnel.
The results were positive as most evaluation models improved significantly after training on AEC data sets. In addition, training models on AEC data increased the performance of clinical electronic ECG systems during pre-hospitalization screening of individuals with suspected acute cardiac syndromes. autoencoders can effectively identify changes over time by comparing archived versions of the same data set. This makes them uniquely suited for healthcare applications, as doctors and healthcare professionals deal with patient data sets that evolve over time.
Medical professionals can use autoencoders to diagnose various health issues by examining historical medical records. For example, autoencoders could be used to assist in the development and evaluation of personalized health plans. These plans are designed to address the specific needs of individuals based on their health history. In addition, autoencoders are being used to improve disease prevention through early detection. This is accomplished by identifying patterns in data and predicting future events- such as heart attacks or cancer growths- based on historical data sets.
Autoencoders have also proven useful outside of healthcare applications. For example, they’re being used to train computer vision algorithms to identify objects in photographs. This is useful in fields such as automotive or industrial maintenance where staff members must identify equipment or documents for maintenance purposes. In addition, autoencoders are being used to train algorithms for game theory and AI development by identifying optimal strategies for decision making under uncertainty. These uses demonstrate how versatile the technique is and suggest how far it’s already come in just a few years.
Autoencoders have a lot of potential in healthcare applications- from early diagnosis to training computer vision algorithms. The recent developments in the field suggest that this technology is still in its infancy, but it’s exciting to think about how this technology will change the way we interact with the medical system.
Autoencoders for risk assessment and treatment recommendation
Autoencoders have been used by healthcare institutions for risk assessment and treatment recommendation. For example, an autoencoder using CODA feedback for left atrial hypertrophy detection trains an AI algorithm to identify patterns in electrocardiogram (ECG) waveforms associated with atrial enlargement in patients with atrial fibrillation (AF). After training, healthcare providers can use the algorithm to predict atrial fibrillation and analyze patient ECGs remotely. Autoencoders are especially useful in this application when trained with patient-level clinical data such as age, sex, and medical history. This enables autoencoders to better target patient care and reduce unnecessary testing and treatment-related complications.
Anomaly detection
Autoencoders exploit the properties of neural networks in a special way to enable some efficient ways of training networks to learn normal behavior. When anomalous data points arrive, autoencoders cannot encode them well. It has learned to represent patterns that are not present in that data. When trying to reconstruct the original data from its compact representation, the reconstruction is not similar to the original data. This helps to detect anomalies as they occur. The goal of such a process is to attempt to reconstruct the original input from the encoded data, which is crucial when building an anomaly detection engine.
Autoencoders are a powerful tool for patient data analysis
Last but not least, autoencoders are a very powerful anomaly detection tool. Through the process of encoding and decoding, you know how well you can usually reconstruct your data. If the autoencoder is then fed anomalous data, showing something the model has never seen before, the error in reconstructing the input after the bottleneck will be much higher.
Autoencoders provide an effective way to greatly reduce input data noise, making deep learning model building more efficient. They can be used to detect anomalies, solve unsupervised learning problems, and remove complexity from datasets.
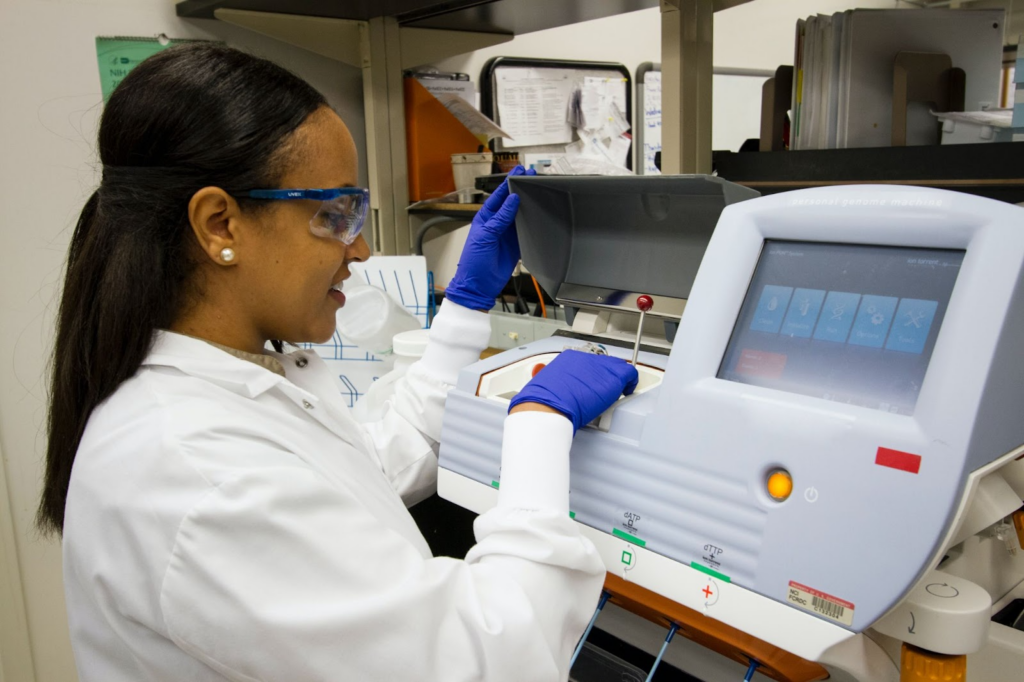
Autoencoders are a variant of artificial neural networks that learn distributed representations of their inputs
They learn parameters to transform the data into a hidden layer and then reconstruct the original input. By using hidden layers that are smaller than the number of input features, or “bottleneck” layers, autoencoders are forced to learn key patterns in the data 9. To avoid over-reliance on certain features, two techniques are generally used. In denoising autoencoders, noise is added to the corrupted part of the input 9,10. Alternatively, a technique called dropout removes random entities and connections from the network, forcing it to learn to generalize11. Autoencoders have been shown to produce useful higher representations in both simulated and real EHR data. Because autoencoders learn by reconstructing the original input from corrupted versions, imputation is a natural extension 12, 13 .
Conclusion
Autoencoders are a machine learning technique that assist in the identification and treatment of diseases. Autoencoders are also used to interpret complex data sets and train computer vision algorithms. Autoencoders are an innovative form of AI used in several important fields of research- including communication, defense, and healthcare. This technology has many applications in these fields due to its ability to effectively train machine learning algorithms to detect heart conditions. Furthermore, autoencoders can be used to encode and decode data with error correction capabilities for secure communication These applications suggest the technique is still in its infancy and has a lot of potential. Healthcare providers have successfully used autoencoders in their practices thanks to these innovative applications!
Sources
1. https://www.mdpi.com/2076-3417/12/10/4931
2. https://www.frontiersin.org/articles/10.3389/fgene.2021.607471/full
4. https://rapidminer.com/blog/autoencoders/
5. https://rapidminer.com/blog/autoencoders/
6. https://www.ncbi.nlm.nih.gov/pmc/articles/PMC5144587/
التعليقات